This AI model can help catch kidney damage, but it works less well in women
Medical AI has been in the headlines (including our own) quite a lot recently.
ChatGPT has taken our industry — and the whole world — by storm. The number of AI-enabled medical devices is increasing. AI medical startup funding also continues to grow.
This week, we were excited to read a new study in Radiology that demonstrates how AI can help triage people reporting to the ER with acute chest pain.
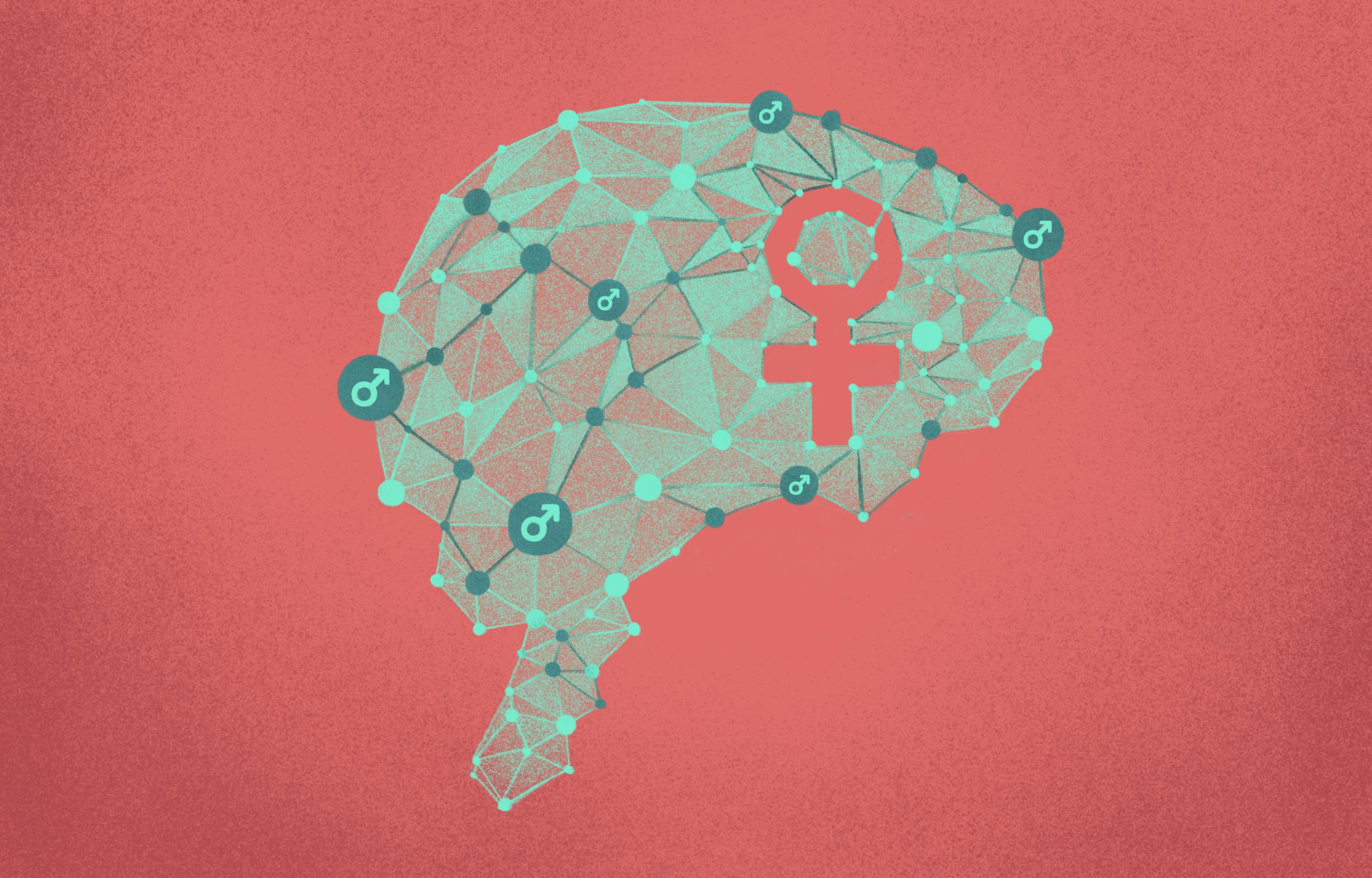
Medical AI has been in the headlines (including our own) quite a lot recently.
ChatGPT has taken our industry — and the whole world — by storm. The number of AI-enabled medical devices is increasing. AI medical startup funding also continues to grow.
This week, we were excited to read a new study in Radiology that demonstrates how AI can help triage people reporting to the ER with acute chest pain.
Researchers found that deep learning analysis can be applied to chest x-rays of emergency department patients reported acute chest pain to determine which patients were at high risk of a cardiac event or death. The next step is to validate the findings on external datasets beyond the study’s 23,000 chest scan sample to see how it can improve real-world triaging.
However, another recent study has shown that generalizing real-world functionality from limited datasets is exactly where medical AI can run into trouble.
Namely, a lack of demographic diversity in training datasets can make it difficult for AI tools to be useful for all patients.
Read on for more on what happened with this study.
The original model: predicting kidney damage with a VA dataset
Google researchers originally demonstrated that an AI system could accurately predict acute kidney injury up to 48 hours in advance.
This condition is a leading killer of hospitalized patients, so the use of such a model in the field could potentially save a lot of lives.
Naturally, this news was met with a lot of enthusiasm.
The Department of Veterans Affairs — whose de-identified patient database the model was trained on — said in 2019 that the promising results meant it would immediately start work towards bringing the tool into practice.
However, in their paper, Google researchers did mention performance issues in women, emphasizing the need for more testing.
This is because the VA’s dataset was made up of veterans, who were predominantly male — 94% male, to be exact.
The new study: finding performance issues in female patients
This month’s study on the model, published in Nature, demonstrates that this model is not as generalizable as the VA had hoped. In fact, the study authors emphasized the performance issues are greater than the initial paper even flagged.
The University of Michigan researchers conducted their evaluation with a replica of the original AI system. They addressed the original VA datasets male skew by retraining the model using sex-balanced data from Michigan and VA facilities.
Doing so, they showed the model, as feared, does not perform as well on women. It overestimates the risk for some women and has less accuracy for female patients overall.
“If we have this problem, then half the population won’t benefit,” said lead author Jie Cao.
The authors refer to this model as an example of the “artificial intelligence (AI) chasm,” or “high-performing AI models that fail to reach the bedside due to challenges involved in real-world implementation.”
They also point out that a lack of public availability of many such models (including this one) make reproducibility a chronic problem for the progress of clinical AI. How else can we as an industry get them ready for real-world applications?
Why this matters: we need diversity in research data
By now, it should be clear: AI can improve survival odds for ER patients and decrease preventable deaths in hospitalized patients. It’s an amazing technology that can change healthcare for the better.
However, for it to have these impacts we dream of, we need to train models on demographically-diverse datasets.
Beyond AI, diverse demographic representation in research studies at large is vital for technology we develop to be actually useful — and safe to use — for a general patient population.
As this week’s Pulse Check interviewee, femtech attorney Bethany Corbin, points out, women’s exclusion from most clinical trials until the 1990s is still having repercussions to this day. The medical literature holds less information about how many disease presentations and therapeutics operate differently in female bodies.
For more on our thoughts here, we’ve discussed this issue at length in our piece on integrated clinical trials. We see this research approach as a promising sign the industry’s recognizes the importance of diverse research datasets.
And, of course, as Cao, et al. urge: We need to increase access to AI models for a greater number of researchers.
Reproducibility is a core tenet of scientific research for a reason. We need to prioritize the confirmation of results we all get (rightfully) initially excited about to be able to bring innovations to the patients and providers who need them most.
That important scientific work — even when, as in this case, it brings us disappointing results — should be celebrated as well.